This blog post is based on the webinar of the same name, recorded on Tuesday, January 25, 2022. The webinar featured Abdul Rehman (SSIMWAVE), Jose Jesus (Conviva), Thierry Fautier (Harmonic), and Thomas Guionnet (Ateme). It was moderated by Streaming Video Alliance Executive Director Jason Thibeault.
The technology behind streaming is evolving, just as broadcast is evolving to streaming. As software development transforms from siloed, monolithic architectures to scalable, containerized microservices, new opportunities for enhanced functionality within the streaming workflow are appearing. Whether that’s employing AI and ML and the edge or implementing automation, such as ticket generation based on exceeding KPI thresholds, the future of the streaming technology stack seems to have no limits. With that in mind, it is important to explore the state of some key technologies, such as AI, ML, and Edge Compute, and how they might be applied in the streaming workflow to improve operations, resiliency, and the viewer experience.
Challenge #1: Integration into Streaming Workflows
One of the challenges we face is integrating AI and ML processes into streaming workflows while minimizing the cost. While we save bandwidth and maintain the video quality on pre-recorded video based on in-line decision-making, it is much more difficult to apply to live services. When streaming Live TV, compression can result in additional latency since the video stream is streaming in real-time and costs significantly more CPU. For situations where we see spikes in traffic on live services such as the World Cup or the Super Bowl, it may be necessary to make the decision to reduce resolution for, say, mobile viewers. But, in many cases, improvements to compression and latency are often ad-hoc procedures. Utilizing AI and ML to learn these procedures and replicate them later can help improve the experience of future live events without the “trial and error” approach of ad-hoc approaches. Applying AI and ML to compression in this manner ensures a more efficient approach as the algorithms are tuned prior to implementation.
Challenge #2: Balancing Viewer Experience and Network Needs
Another challenge for integrating AI and ML processes is the balance between network needs and viewer experiences. Overall, network needs should be taken into consideration, since networks such as mobile versus fiber have different components and constraints on their bandwidth. However, perception quality is difficult for automated systems, but is what we are looking for to optimize streaming. In this case, AI has been useful to help design video encoders when the goal is to maximize video quality within certain constraints. AI can be used to help with modeling human perception and is an area for further exploration as we continue to look for practical solutions for both network and viewer needs.
Challenge #3: Accurate Training and Fine-Tuning Systems
The question that is constantly revisited in the development of AI and ML systems is how to accurately train them in a way which is also part of the first challenge we discussed. It can be difficult to optimize AI and ML systems if the scope of the optimization is too broad. The ideal approach is to find the right fit for the ML application (in the specific problem that you are trying to solve), rather than applying it to everything. Even if fine-tuning is not the main issue, there is also the cost of implementing AI based heavy algorithms. A heavy algorithm, which can represent a large code base or require extensive CPU resources, may have a ripple effect. Sure, it can optimize the content, but it could cost more to use and also increase latency if it is employed in a real-time capacity. For example, consider variable frame rate. If an AI or ML algorithm is trying to optimize this during the encoding process, and it breaks, it could result in subscriber attrition and cost additional money to refactor or optimize. There are also issues with running it live, where it may run fine on one system but not on another. This can require the need for additional fine-tuning of the algorithm on specific hardware needs.
Bringing AI and ML Integrations into the Mainstream
What might we see moving forward? There are techniques coming from the gaming world being explored for reducing CPU load and frame rate for certain streaming constraints. Through the development of different AI and ML use, there are new ways to handle that video stream that were not possible before. Dynamic resolution encoding is also being adopted in specific use cases to improve compression efficiency. Additional developments, as the industry continues to test these technologies in use cases like live sports, may include the deployment of smart resource applications to optimize quality on the fly based on available network and client resources.
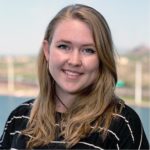
Sydney is a freelance writer working with the Streaming Video Alliance to develop blog posts and other content.
-
Jason Thibeault
-
Jason Thibeault
-
Jason Thibeault